Enabling Human-Machine Teams Starts with Modelling their Interactions using a Standard Vocabulary-A Set of Information Tokens!
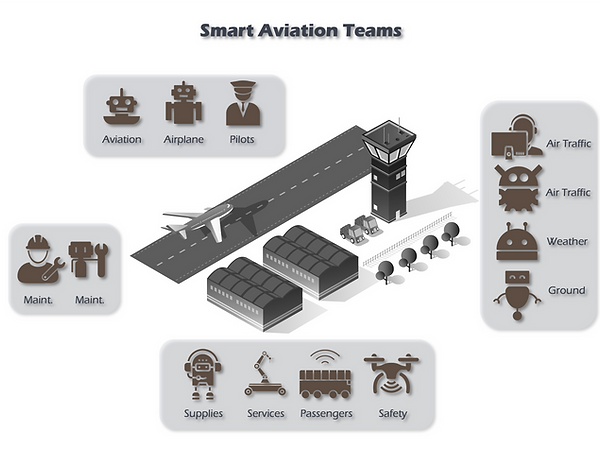
Digital Twins for Human-Machine Teaming (HMT)
Human-Machine Teaming (HMT) refers to situations in which people and intelligent agents, or robots, work together to achieve a common objective.
It is projected that managing the increasing complexity of many domains such as aviation and smart cities can be only achieved through human and intelligent agent teams.
Such teams would consist of-for example-pilots, air traffic controllers, and ground staff working together with aircraft agents, air traffic control agents, weather agents, maintenance agents, smart drones, and self-driving ground vehicles.
In an effective HMT, and given a shared objective and task environment, each team member must be able to identify the actions it can take which will enable the entire team to accomplish the shared objective.
HMT Challenge-Defining a Shared Task Model
Each human team member, such as a sports team, has a mental model of the game's rules, the playing field, and their colleagues' roles (the task environment). Then, each player uses their own model to "simulate" numerous interaction scenarios and identify the ideal course of action for a specific circumstance.
Even though each player follows the same game plan and objectives, if there mental models are not consistent (i.e., they have different interpretations of the game rules or the roles of other players) then it is highly unlikely that their actions are consistent with those of their teammates. Accordingly, the team will be ineffective in achieving their objectives.
​
Thus, the greatest obstacle to attaining effective HMT is providing each team member with a consistent model of the Task. In addition, the task model must be updated in real-time to reflect the task progress, thus allowing each team member to base their actions on the same task status, which increases the team's efficiency significantly.


The HMT Platform-Providing a Virtual Task Environment
The primary objective of the HMT Platform is to provide each team member with an up-to-date model of the task they support. Each team member can then use the model to choose which actions to take based on their role. Each team member's actions, in turn, update the task reference model and accordingly influence the following decisions of all other team members.
The task reference model is the Information Heat Map (IHM). The IHM is a data model representing a task (or scenario) in terms of the many input and output parameters defining it, their correlations, and conditional dependencies.
Using the IHM as the environment, each team member can then use reinforcement learning to determine the optimal course of action for attaining the team's objectives. The IHM thus provides a virtual task environment, the task reference IHM, in which all team members communicate and are connected in real-time, thus enabling an effective HMT.
How is the HMT Platform Different?
The HMT Platform is initiated by defining the scenarios capturing the various tasks as information models, the Information Heat Maps, IHMs. A member's unique IHM combines one or more Reference IHMs to cover the asks the member needs to support.
​
The IHM's most significant innovation-and difference-is capturing scenario parameters as a range of discrete values. For example, temperature is recorded in discrete value ranges: 35-37, 38-40, or > 40. A measured value is then represented as a "1" for the matching range and a "0" for other values.
​
The discrete representation of data enables the HMT Platform to capture, describe, and correlate any metric type in a single, consistent structure: the IHM. In other words, an IHM can model and represent a multitude of complex tasks or scenarios as a single-task representation. Team members can then decide what to do based on the same, up-to-date representation, which they can access through their own apps (HDT/IDT)..


Why is the HMT Platform a Better Solution?
The HMT Platform and the connected HDT/IDT Apps create a virtual task environment similar to that of multiplayer online games. According to this analogy, a team member's HDT/IDT stands for the avatar with which they interact with the environment. In addition, and as in online games, only that part of the environment is rendered which is relevant to a player, i.e., each team member's IHM represents only that part of the environment that is relevant to their role or task. .
​
On the other hand, scenario and task owners can manage a complete team's tasks by modifying the parameters of the relevant IHMs, which then propagates through all relevant HDTs/IDTs and accordingly updates their subsequent decisions.
​
Finally, mature, role-specific HDTs/IDTs can be assigned to new team members to speed their learning and adaptation to their roles, ensuring task performance consistency.
Building Human-Machine Teams-How to Start?
As with other HDT/IDT use cases, building digital twins-enabled HMT environment starts by identifying a set of tasks and scenarios to be carried by the HMT team and then build a prototype of the various involved roles to ensure the feasibility of the HMT Platform.