Patient-Centric Predictive Solutions Start with Establishing a Common Vocabulary for Medical AI!
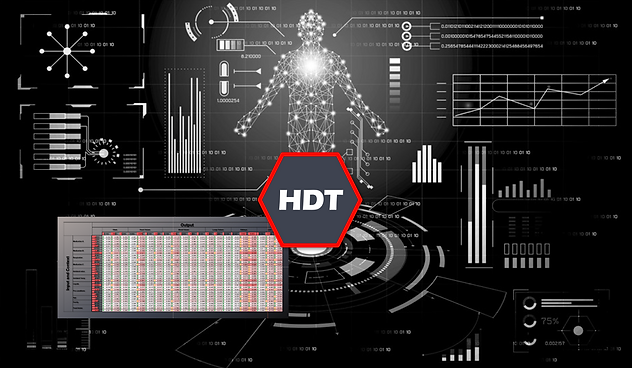
Patient-Specific Predictive Diagnostic
Even tiny changes in patient vital signs and measured values can signal a developing problem. Time is of the essence when it comes to predicting these deviations, placing them in context, understanding them, and reacting to them with a therapeutic change. These tasks are especially demanding for physicians who have little experience with the patient group.
Challenges with ICU Care
-
No reliable technology for predicting ICU patients' critical events
-
No patient-specific predictions or risk alarms
-
Critical ICU events may have long-term effects on patients
-
Patient risk assessment is still dependent on the attending physician's competence and knowledge, which may vary between shifts and facilities
-
Many critical events may be predicted if there is real-time, comprehensive patient monitoring
-
Longer Mean Time to Respond (MTTR) after identifying a critical event (assessment, data analysis, treatment option assessments) raises patient risk
-
High treatment cost and limited ICU capacities
The Patient-Human Digital Twin (Patient-HDT)
-
Provides automated and patient-specific predictive risk notifications
-
Provides treatment recommendations and simulations
-
Provides consistent and expandable knowledge base to close the knowledge gap across medical teams
-
Compensates for the increasing lack of specialized medical personnel
-
Expandable to cover various types of patient metrics and treatments
-
Open architecture that can integrate with, and learn from any patient-related data source
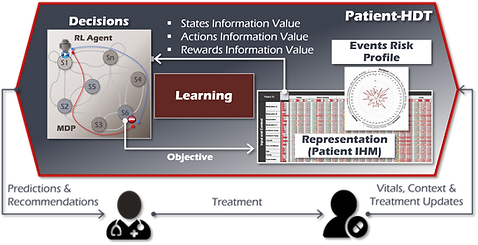
How is the Patient-HDT App Different?
-
Patient-specific, can be provided as a service to patients to improve risk prediction after they return to their everyday life
-
Continuous learning from patients’ digital interactions to enrich risk assessment and recommendations
-
Continuous and automatic updating with new medical information to improve risk predictions and mitigation recommendations
-
Insights from diverse Patient-HDT can be incorporated into a single dynamic medical knowledge base to use anywhere to enhance treatment quality
Start with the Patient-HDT Feasibility Assessment
Building data-driven healthcare solutions is a challenge. Not only because of patient data privacy, but also because of the extensive specialized medical expertise required to engineer this data and provides insights from it.
Our method uses raw data to define initial data-related insights to determine the feasibility and value of the Patient-HDT.
​
The first step is identifying a group of critical conditions or risks that significantly impact treatment. Next, a set of parameters and metrics associated with the conditions or risk is identified.
The data associated with the parameters and metrics in scope is then obtained from historical health records. The Patient-HDT uses the obtained data to learn the information model particular to the conditions or risks under consideration (Information Heat Map, IHM).
The IHM provides initial insights into the correlations of the factors impacting a condition. Further, the IHM allows the medical team to gain insights into how controlling specific parameters would impact the condition or risk of a patient.
​
Validating such insights is the first step towards validating the value and feasibility of the Patient-HDT!
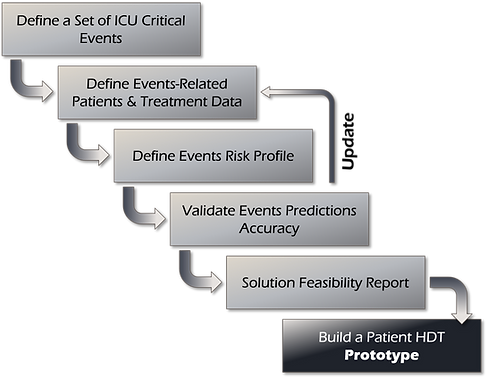
Events Risk Profile Example
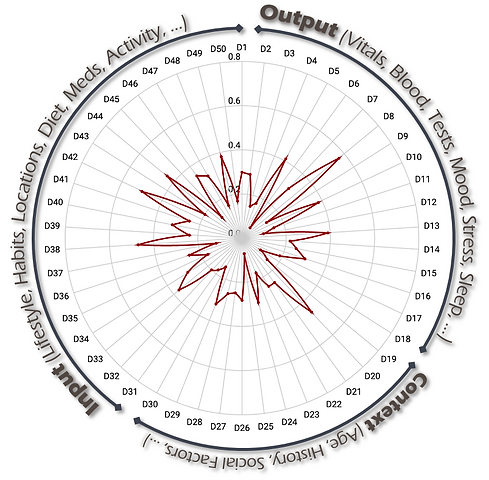
Modeling Patients, A Key Challenge for AI Solutions in Healthcare
Digital twins are expected to offer unprecedented insights into a variety of fields of medicine and healthcare. At some point, digital twins will fundamentally alter how physicians engage with their patients. Doctors would employ digital twins to diagnose patients' conditions more accurately, simulate treatment possibilities, and provide assistance during life-saving surgery.
​
On the other hand, the human body is an extraordinarily complex and dynamic system. Constructing a model of an organ or a patient remains a complex undertaking, made even more challenging due to the sensitivity around patients' health data.
​
However, utilizing the Patient-HDT Platform enables a relatively simple and staged introduction of digital twins into the medical sector. For example, the radar chart depicted here, which models a hypothetical medical risk event, can provide preliminary insights into the significance of several medical and lifestyle factors associated with that risk event and their likelihood of contributing to that event, all without application of elaborate machine learning techniques.
​
Once the model have been confirmed for its consistency, accuracy, and value in terms of risk reduction, the more elaborate implementation of the Patient-HDTs for the individual patients can commence.
Patient-HDT Data Structure
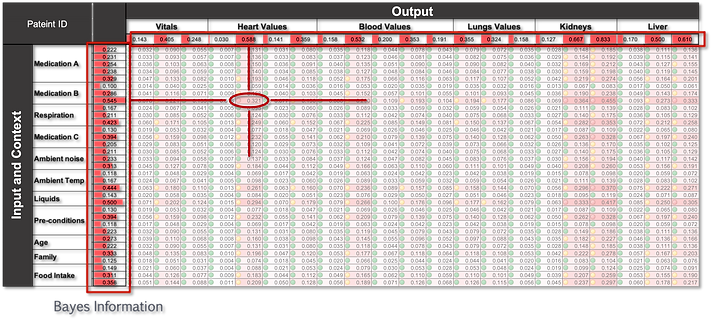
The patient Information Heat Map (IHM), which captures all pertinent patient and treatment data, is the central component of the Patient-HDT. The Patient IHM is initiated using historical patient data. A cell in the heat map depicts, for instance, the degree or association between a particular dosage of a particular medicine and a particular heart rate range. Using Bayesian inference and the same set of historical data, the Patient IHM determines, for a given risk event (any combination of output values), the correlation of each input on that risk event. These values are then used by the Patient-HDT to build the Event risk profile, to determine the onset of critical events and recommend courses of actions to mitigate the risk.